Digital Twin and Parameters Identification approaches for optimization of production lines
Any company that doesn’t constantly optimize their production processes risks being surpassed by a competitor that has innovative solutions in production. Considering the rising cost of energy, the need to generate profits for manufacturers becomes even stronger.
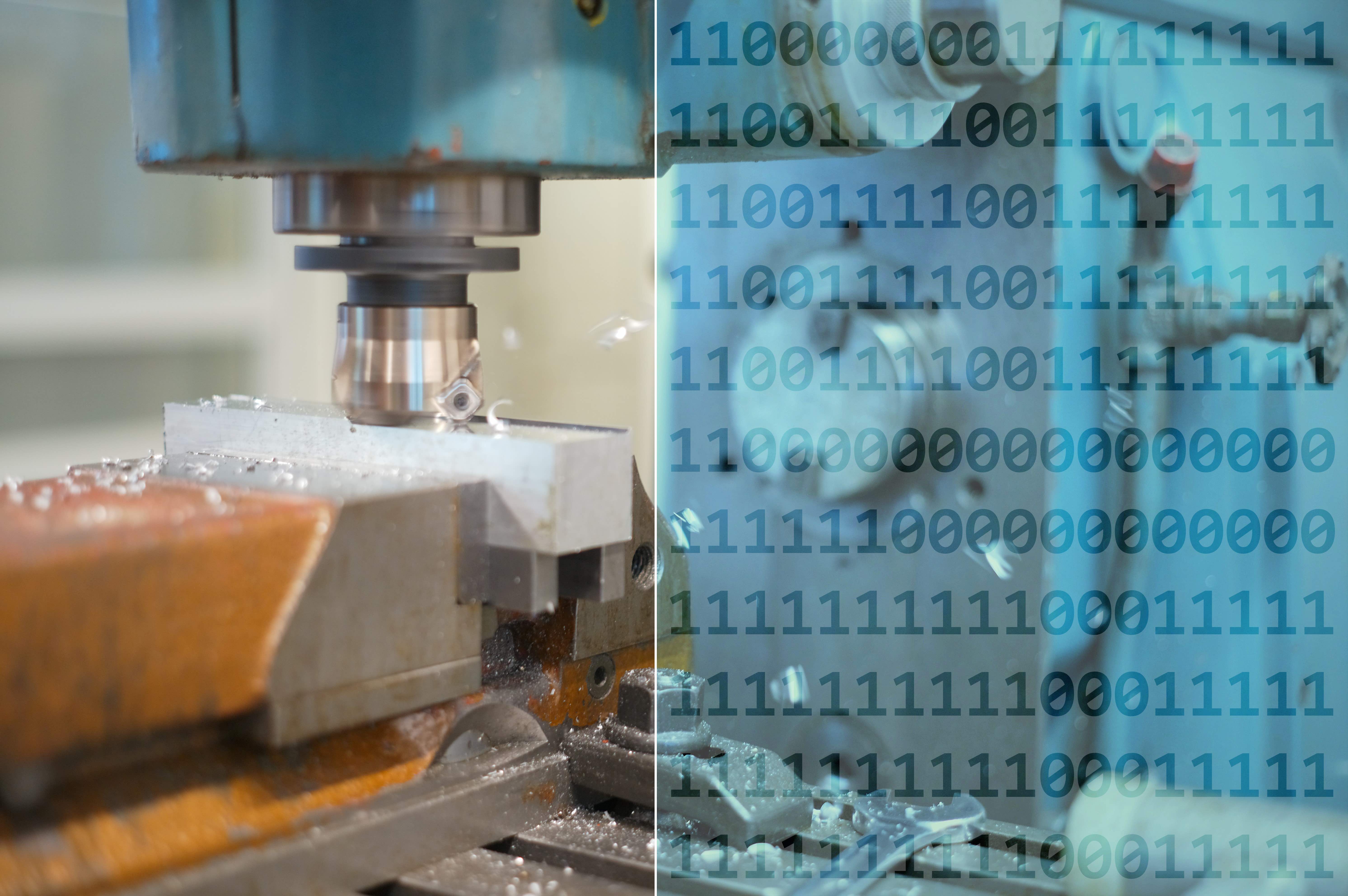
Background
To succeed in today's marketplace, companies must implement different production optimization strategies. Production optimization are the approaches to maximization of production at a given factory using existing equipment. It involves improving the operating parameters of a factory or facility to increase margins without having to upgrade the facilities themselves. Thus, development approaches to optimization of Production Lines (PL) with use of computer-aided and AI-based smart solutions is of vital importance for all manufacturers. The main goal of the project can be formulated as the development of an approach to optimize the production line by creating a digital twin (DT) of the line and identifying its parameters based on the AI.
Challenge and tasks
The main task of the project is to develop a model to predict the performance of the PL, allowing to optimize its parameters according to various criteria of efficiency, and to build a model to evaluate the performance of the PL. To achieve this goal, we will need:
- Develop a digital twin (DT) of a given production line capable of running simulations and creating all the necessary parameters for (at least) a production cost model.
- When designing a DT, special attention should be paid to machining operations, taking into account different process variations and the human factor.
- Find optimal parameters of the PL using conventional approaches and AI-based.
- Generate data influencing the performance parameter(s) and build the model by identification of the system (PL) parameters using AI.
Reporting
The work is suitable for 1-2 students with a background in manufacturing systems, moderate knowledge of Matlab/Python and basics of Machine Learning.
The thesis will be concluded with a written MSc thesis and oral presentation will be given at LTH, LU.